Why Do Most AI Projects Fail? Uncovering the Challenges and Solutions
Top 14 reasons why AI/Machine Learning Projects fail.
In today’s fast-paced technological landscape, artificial intelligence (AI) has emerged as a transformative force with immense potential. However, the journey from conception to successful implementation of AI projects is often riddled with challenges, leading to a high failure rate. In this article, we delve into the reasons behind the failure of most AI projects and explore actionable strategies to overcome these hurdles.
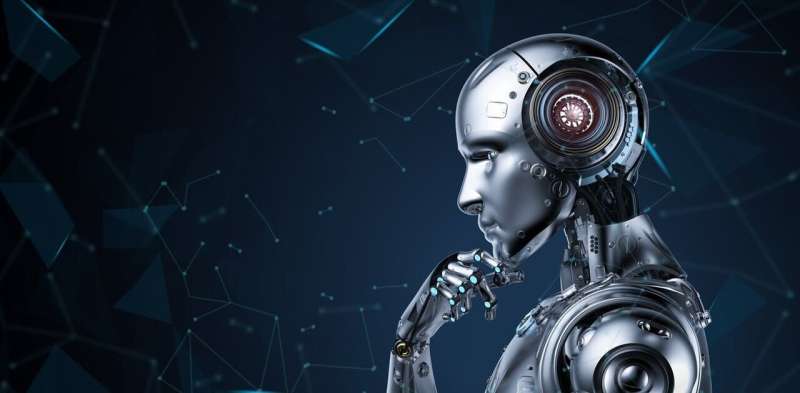
AI has been hailed as a game-changer across industries, promising automation, enhanced decision-making, and innovation. However, the landscape is strewn with the remnants of AI projects that have fallen short of their objectives. Understanding the common pitfalls can pave the way for successful AI implementations.
Lack of Clear Objectives and Planning
A cardinal sin in AI project development is embarking without well-defined objectives and a robust plan. Without a clear roadmap, projects lose direction and fail to deliver meaningful results.
Setting Vague Objectives: The Case of Chatbot Blues
The lack of well-defined objectives was evident in a chatbot project by Company X. The project aimed to enhance customer service but failed due to vague goals. The chatbot lacked direction, leading to customer frustration and abandonment.
Insufficient Data Quality and Quantity
AI thrives on data, and lack of high-quality, relevant data can cripple even the most sophisticated algorithms. Garbage in, garbage out—a foundational principle that underscores the importance of data quality.
Data Deficiency: The Self-Driving Car Setback
Company Y’s self-driving car project stumbled due to inadequate data. The algorithms couldn’t comprehend complex traffic scenarios, resulting in accidents. This serves as a stark reminder that data quality is the bedrock of AI success.
Complexity of Model Selection
Selecting the right AI model is akin to choosing the right tool for a task. The plethora of options can lead to decision paralysis, or worse, selecting an ill-fitting model that yields subpar results.
Model Mismatch: A Misstep in Image Recognition
In the world of image recognition, Company Z’s failure looms large. They selected an unsuitable model for recognizing rare species of birds. The AI struggled, misclassifying species, and ultimately eroding trust in its capabilities.
Inadequate Expertise
AI demands a specialized skill set encompassing data science, machine learning, and domain knowledge. A shortage of skilled professionals can lead to faulty development and execution.
Skills Shortage: The Shipping Recommendation Fiasco
When Company A ventured into AI-powered shipping recommendations, they lacked the requisite expertise. The algorithm couldn’t optimize routes efficiently, leading to increased costs and shipping delays.
Ethical and Regulatory Concerns
As AI becomes more integrated into daily life, ethical considerations and regulatory compliance become paramount. Ignoring these factors can lead to public backlash and legal issues.
Ethical Quandaries: Social Media Content Moderation
Company B’s AI content moderation tool made headlines for the wrong reasons. It failed to distinguish between hate speech and legitimate content, highlighting the critical importance of ethical considerations in AI projects.
Scalability Issues
AI projects often struggle to scale beyond pilot phases. Inadequate infrastructure and planning for scalability can render projects obsolete once they encounter real-world demands.
Scaling Struggles: The Virtual Shopping Assistant
Company C’s virtual shopping assistant was a hit during trials but faltered when scaled. The infrastructure couldn’t handle increased user loads, resulting in crashes and missed sales opportunities.
Integration Challenges
Integrating AI systems with existing technologies and workflows can be a logistical nightmare. Compatibility issues and disruptions to established processes hinder successful implementation.
Integration Woes: ERP System and AI Misalignment
When Company D integrated AI with their Enterprise Resource Planning (ERP) system, chaos ensued. Incompatible interfaces and data mismatches disrupted operations, underscoring the need for seamless integration planning.
Changing Business Needs
In the dynamic business landscape, objectives can shift rapidly. AI projects that don’t adapt to changing needs become irrelevant and fail to deliver value.
Adapting to Change: The Healthcare Diagnosis Dilemma
A hospital’s AI-driven diagnostic tool hit a roadblock as it couldn’t adapt to evolving medical practices. Failing to incorporate the latest research made the tool obsolete and potentially dangerous.
Unrealistic Expectations
The hype surrounding AI can lead to inflated expectations. When reality falls short of these expectations, projects are prematurely labeled as failures.
Unrealistic Expectations: The Language Translation Flop
Company E aimed for AI-powered instantaneous language translation but fell short. Expectations of near-perfect translations didn’t align with reality, leading to disillusionment.
Communication Gaps
Effective collaboration between technical and non-technical stakeholders is crucial. Miscommunication can result in misaligned objectives and eventual project failure.
Communication Breakdown: Robotics in Customer Service
Company F’s attempt to implement robots in customer service led to chaos. Lack of communication between the robots and human staff resulted in confused customers and compromised experiences.
Resource Constraints
AI projects require substantial resources, including time, money, and talent. Underestimating these requirements can lead to project abandonment midway.
Resource Crunch: AI-Powered Inventory Management
Company G underestimated the resources required for their AI inventory management project. The algorithm couldn’t handle the vast data influx, resulting in inaccurate stock levels and frustrated customers.
Resistance to Change
Introducing AI often disrupts established processes and can be met with resistance. Overcoming this resistance requires strong change management strategies.
Resistance to Innovation: AI Time Tracking Troubles
When Company H introduced AI-based time tracking, employees resisted the change. The lack of proper change management led to inaccurate time logs and a dip in employee morale.
Technical Debt
Cutting corners in development to expedite timelines results in technical debt. This accumulated ‘debt’ can lead to system breakdowns and project failure down the line.
Technical Debt Debacle: Financial Fraud Detection
In the pursuit of quick deployment, Company I accrued technical debt in their fraud detection AI. Over time, the system’s performance deteriorated, missing crucial fraud instances and tarnishing their reputation.
Lack of Continuous Monitoring and Maintenance
AI is not a one-time implementation; it requires ongoing monitoring, maintenance, and updates. Neglecting this aspect can lead to degradation in performance and eventual failure.
Neglecting Maintenance: AI-Powered Language App
Company J’s language learning app was a hit initially but lost its charm over time. Neglecting regular updates and improvements led to stale content and reduced user engagement.
Conclusion
The landscape of AI projects is littered with failures that hold valuable lessons. From planning and data quality to expertise and ethics, addressing these challenges is crucial for successful AI implementation. By recognizing and mitigating the factors that lead to failure, organizations can harness the true potential of AI and drive meaningful transformations.
Frequently Asked Questions
Q1: Are AI projects inherently doomed to fail?
A1: No, AI projects have immense potential for success, but they require careful planning, expertise, and continuous effort.
Q2: How can businesses ensure data quality for AI projects?
A2: Businesses should invest in data collection, cleansing, and validation processes to ensure high-quality data inputs.
Q3: What role does executive buy-in play in AI project success?
A3: Executive support is crucial for securing resources, aligning objectives, and overcoming organizational hurdles.
Q4: Can small businesses embark on AI projects successfully?
A4: Yes, but they should start small, focus on specific objectives, and collaborate with experts if needed.
Q5: What’s the future of AI project management?
A5: The future involves more streamlined integration of AI, increased focus on ethics, and enhanced cross-functional collaboration.
Machine Learning books from this Author: