In the realm of artificial intelligence (AI), generative AI stands out as a fascinating field that has gained significant attention in recent years. This blog post aims to provide you with a comprehensive understanding of generative AI, its history, different types, and how it works. So, let’s dive into the world of generative AI and explore its remarkable capabilities.
Table of Contents
1. Introduction to Generative AI
Generative AI refers to a branch of artificial intelligence that focuses on creating systems capable of producing original and creative content. Unlike traditional AI systems that are programmed to perform specific tasks, generative AI algorithms possess the ability to generate new content, such as images, music, text, and even videos. It utilizes complex neural networks and advanced algorithms to learn patterns from existing data and then generate new content based on that knowledge.
2. A History of Generative AI
The roots of generative AI can be traced back to the early days of AI research. One significant milestone was the development of Markov chain models, which aimed to generate text by analyzing statistical patterns in the input data. However, it was not until the advancements in deep learning and the rise of neural networks that generative AI truly started to flourish.
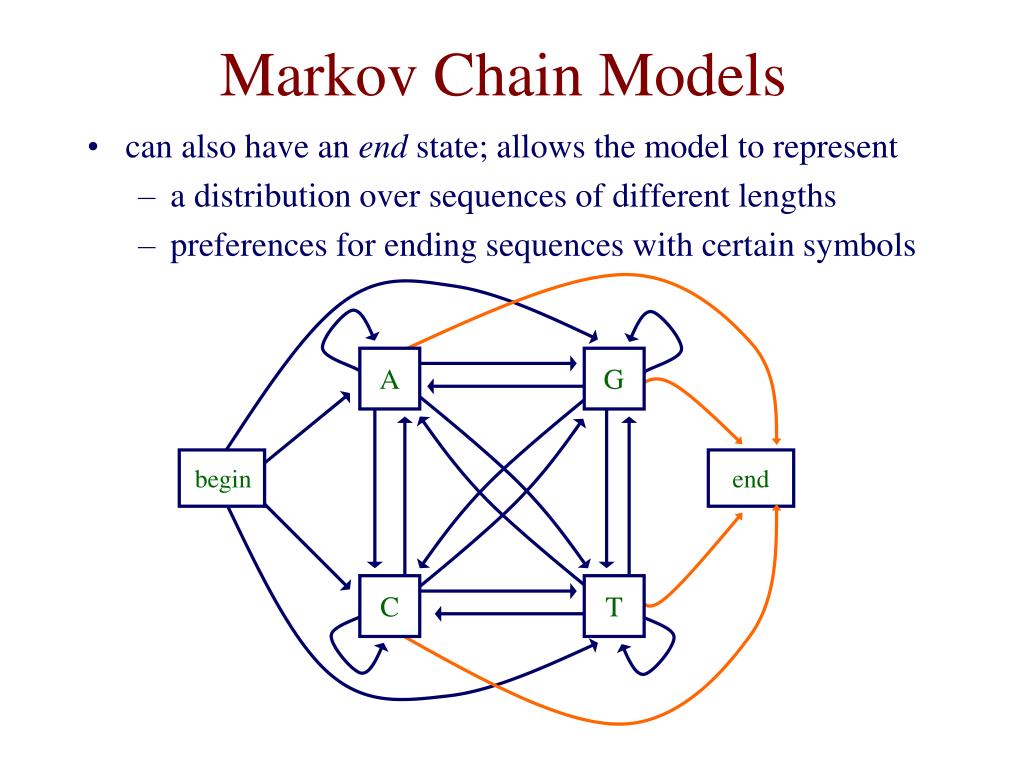
Source: Slide serve
3. Types of Generative AI
Generative AI encompasses various techniques and models, each with its unique approach to content generation. Here are three prominent types of generative AI:
a. Generative Adversarial Networks (GANs)
GANs are a popular class of generative AI models that consist of two neural networks: the generator and the discriminator. The generator network generates content, while the discriminator network tries to distinguish between real and generated content. Through an adversarial learning process, these networks compete with each other, leading to the creation of highly realistic and convincing outputs.
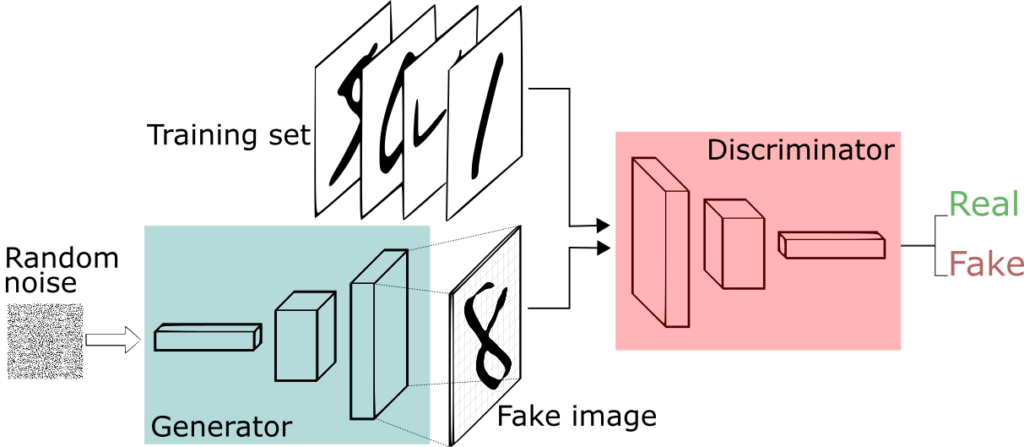
b. Variational Autoencoders (VAEs)
VAEs are another type of generative AI model that leverages both generative and encoding techniques. They are composed of an encoder network that maps the input data into a latent space representation and a decoder network that reconstructs the original data from the latent space. VAEs can generate diverse outputs by sampling from the latent space, allowing for creative exploration and generation.
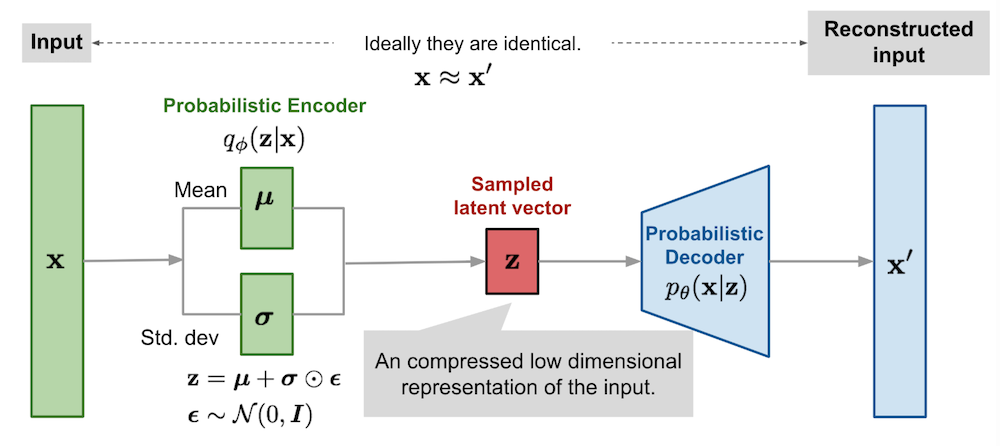
c. Recurrent Neural Networks (RNNs)
RNNs are a type of neural network that excels in handling sequential data, making them well-suited for generative tasks such as text and music generation. They process data in a sequential manner, retaining memory of previous inputs to generate coherent and context-aware outputs. With the help of techniques like long short-term memory (LSTM), RNNs have been successful in generating realistic and human-like content.
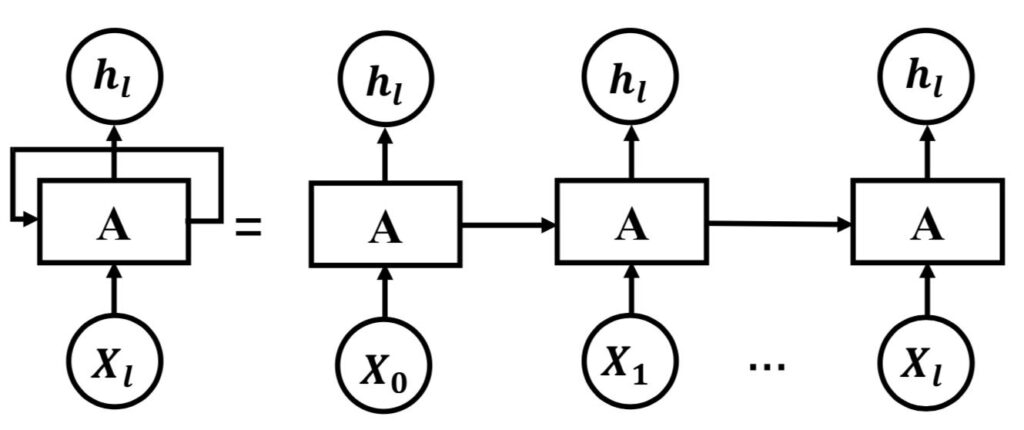
4. How Generative AI Works
Generative AI models learn from vast amounts of training data to capture intricate patterns and generate new content. The general workflow of it involves the following steps:
a. Data Collection and Preprocessing
To train a generative AI model, a significant amount of relevant data is required. This data can be in the form of images, text, audio, or any other type of content, depending on the desired output. The data is then preprocessed, which may involve cleaning, normalization, and transforming it into a suitable format for training.
b. Model Training
During the training phase, the generative AI model learns from the input data to understand the underlying patterns and relationships. This process involves feeding the data into the model and adjusting its
internal parameters through optimization algorithms. The model continues to refine its understanding of the data until it achieves the desired level of performance.
c. Content Generation
Once the generative AI model is trained, it can generate new content based on the patterns it has learned. By providing an input or seed, the model uses its internal representations to generate novel outputs. The generated content can exhibit various degrees of creativity, depending on the complexity of the model and the diversity of the training data.
5. Conclusion
Generative AI represents an exciting frontier in the field of artificial intelligence. Its ability to generate original and creative content opens up new possibilities across various domains, including art, music, storytelling, and more. With techniques like GANs, VAEs, and RNNs, generative AI continues to push the boundaries of what machines can accomplish. As technology advances, we can expect generative AI to play an increasingly significant role in shaping our digital experiences and creative endeavors.
In summary, generative AI is a branch of AI that focuses on creating systems capable of producing original content. It encompasses different types such as GANs, VAEs, and RNNs, each with its unique approach to content generation. By learning patterns from training data, generative AI models can generate new content with varying levels of creativity. As generative AI continues to evolve, we can look forward to witnessing its transformative impact on numerous industries.
Remember, while generative AI holds great potential, ethical considerations and responsible usage should be at the forefront of its deployment.