Artificial Intelligence
What is Chunking in NLP?
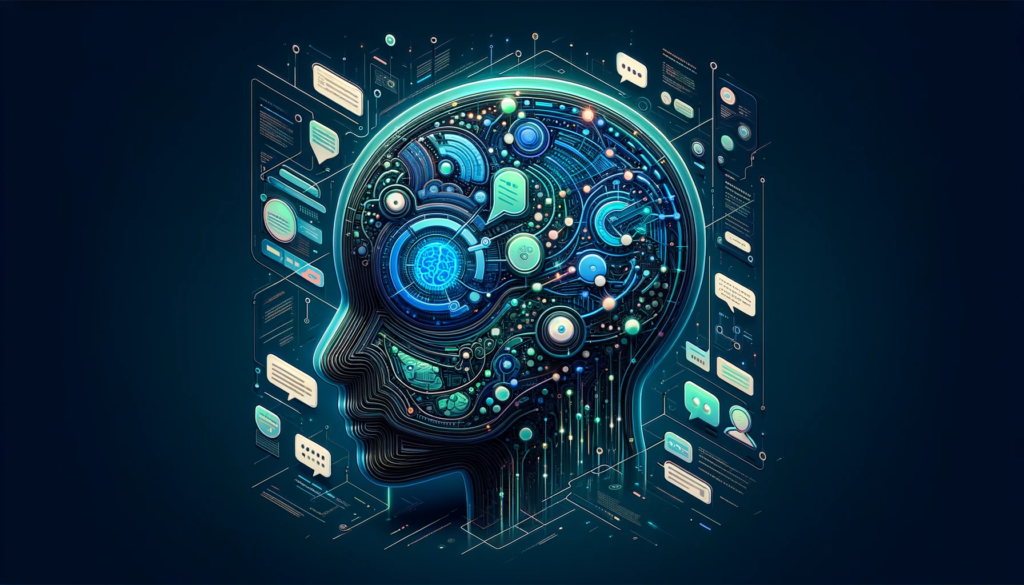
Chunking in NLP is like organizing your messy desk, but for sentences instead of physical objects. Imagine a sentence full of words as a jumble of things on your desk. Chunking helps you group them into meaningful piles, making it easier to understand the sentence as a whole.
Here’s what chunking does:
- Identifies groups of words: Instead of just individual words, it recognizes phrases like noun phrases (e.g., “the blue car”), verb phrases (e.g., “drove quickly”), or prepositional phrases (e.g., “to the store”).
- Improves context: By grouping words together, chunking clarifies how they relate to each other and adds context to the entire sentence.
- Prepares for further tasks: Like sorting items on your desk before tackling a specific task, chunking prepares the sentence for more complex NLP tasks like named entity recognition, sentiment analysis, or even generating new text.
Think of it like this:
- Without chunking: “The cat chased the blue mouse under the sofa.” It’s just a bunch of words.
- With chunking: “The cat (noun phrase) chased (verb) the blue mouse (noun phrase) under the sofa (prepositional phrase).” Now it’s clearer who’s doing what and where.
Here are some applications of chunking:
- Chatbots: Chunking helps chatbots understand the context of your questions and give more accurate and relevant responses.
- Machine translation: Chunking can improve the quality of machine translation by preserving the structure and meaning of the original sentence.
- Text summarization: Chunking can help identify the most important parts of a text and create concise summaries.
Overall, chunking is a fundamental technique in NLP that helps computers understand the meaning and structure of sentences by grouping related words together. It’s like a hidden helper, organizing the building blocks of language to make sense of the bigger picture.